Discussion on some cutting-edge AI technologies
C 2025-05-21
The following is a comprehensive analysis of the cutting-edge technology trends in artificial intelligence for 2025
Brief introduction
The following is a comprehensive analysis of the cutting-edge technology trends in artificial intelligence for 2024, summarizing core technologies and application directions by integrating current technological development dynamics and research achievements:
Common AI Technologies
Small Data and High-Quality Data
Traditional AI relies on massive data to train models, but invalid data may lead to resource waste and model bias. Small data technologies reduce excessive dependence on data through high-precision and highly relevant datasets, enhancing model reliability and generalization capabilities. For example, in medical diagnosis, few-shot learning techniques can train high-precision AI systems using only a small amount of high-quality imaging data.Human-AI Alignment
Ensuring that AI behavior aligns with human values is a core challenge in technological development. By designing reward mechanisms that incorporate ethical considerations, for instance, in autonomous driving, AI must balance efficiency and safety, prioritizing pedestrian protection over merely completing tasks.
AI Ethical Oversight and Usage Boundaries
With the deepening application of AI in fields such as finance and law, establishing ethical oversight frameworks has become necessary. For example, contract review robots use NLP technology to automatically compare legal clauses, avoiding omissions in manual review, while ensuring transparent algorithmic decision-making to prevent abuse.Explainable Models (XAI)
Improving model interpretability enhances user trust. For example, medical AI systems need to show doctors the basis for diagnosis (such as lesion localization) rather than just outputting results, thereby assisting rather than replacing professional judgment.
Large-Scale Pretrained Models
Scaling Law
Increasing the number of parameters significantly improves model performance. Hundreds-of-billion-parameter models like GPT-4 and Gemini have demonstrated excellence in language understanding and multimodal interaction. Studies show that the scaling law is equally effective in image generation (e.g., DALL·E 3) and speech recognition.
Full-Modality Large Models
These models support multimodal data processing, including text, images, audio, and 3D point clouds. For example, robots achieve precise navigation through 3D point cloud data, while full-modality models in virtual assistants can simultaneously parse users' voice commands and gestures.
AI-Driven Scientific Research
Generative AI accelerates research processes. For example, DeepMind’s AlphaFold 3 can predict protein structures and generate experimental plans, shortening drug development cycles.
Embodied AI
Embodied Cerebellar Models
Combining real-time perception with dynamic control gives robots rapid response capabilities. For instance, humanoid robots use multi-model voting mechanisms to perform high-frequency movements (such as grasping fragile objects) in complex environments, with response speeds far exceeding traditional algorithms.Physical AI Systems
Embedding AI into physical devices breaks through traditional functional limitations. For example, industrial robots achieve precise assembly through multimodal perception (vision + touch), while agricultural robots autonomously identify crop diseases and pests and spray pesticides.
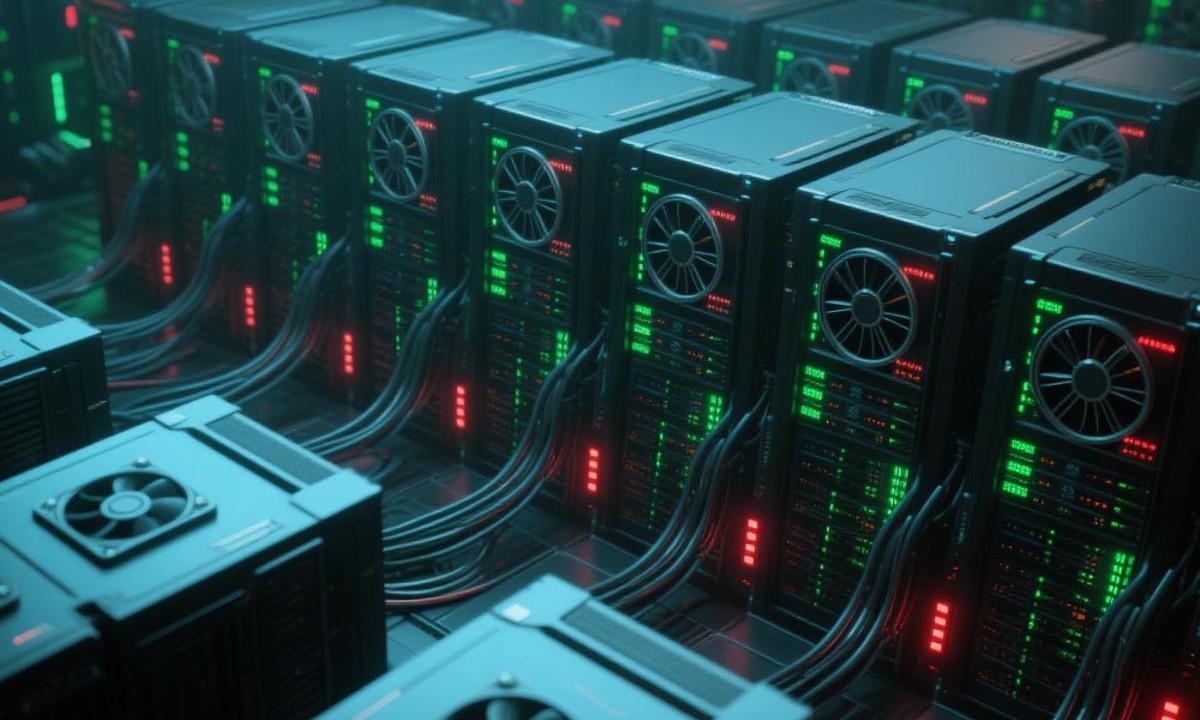
Generative AI
World Simulators
Building highly realistic digital environments for training robots or game development. For example, Meta’s virtual world platform can generate infinite scenario data to accelerate the iteration of autonomous driving algorithms.Cross-Modal Generation Technologies
Examples include Meta’s Seamless model, which supports real-time translation preserving speech emotion and intonation, and Google’s Translatotron 3, which achieves direct speech-to-speech conversion through unsupervised learning, breaking down language barriers.
Breakthroughs in Natural Language Processing (NLP)
Document-Level Relation Extraction: ByteDance’s LogiRE framework combines logical rules with deep learning to extract structured knowledge from long texts (such as identifying human relationships in news), significantly improving semantic coherence.Unsupervised Speech Translation: Google’s Translatotron 3 uses back-translation and speech enhancement technologies to achieve cross-lingual speech conversion without bilingual data, suitable for low-resource language scenarios.
Technical Challenges and Future Outlook
Data Privacy and Ethical Risks: Balancing data utilization and privacy protection, such as promoting federated learning technologies.
Energy Consumption of Ultra-Large Models: Green AI technologies (e.g., model compression, edge computing) have become research hotspots.
Exploration of Artificial General Intelligence (AGI): Combining embodied intelligence with generative AI to drive machines from "task execution" to "autonomous decision-making."
These technological trends not only advance AI applications in scientific research, industry, and healthcare but also profoundly reshape human-AI collaboration models. In the future, technological development must continue to focus on ethics, safety, and sustainability to achieve positive interaction between technology and society. For more detailed cases or technical principles, refer to relevant papers and open-source projects (such as the LogiRE framework codebase).